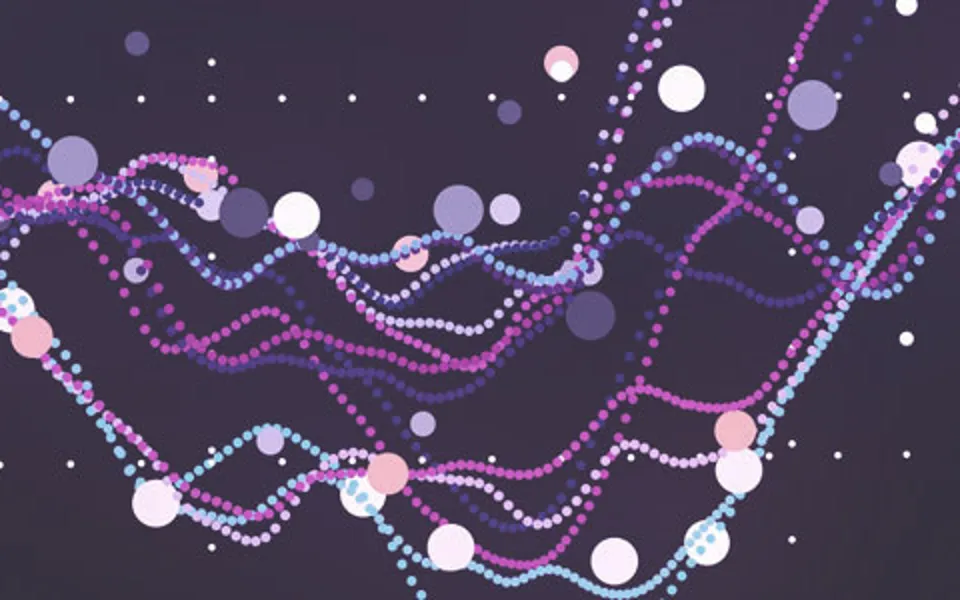
Performance testing of sensor systems – the good, the bad and the hopeless!
01 Jun 2022
In earlier blogs, we showed that sensor performance out of the box couldn’t necessarily be guaranteed to provide data of “reasonable” quality, and that currently, some kind of quality control and data processing is required to improve the data provided.
In this blog, we’ll explore what mechanisms are being put into place to help potential users purchase sensor systems with confidence.
Development of standards for ambient air quality gas sensors
At the end of 2021, the European Standardisation Committee (CEN) released Technical Specification (TS 17660-1) classifications, documenting how to undertake performance tests on gas sensors to classify their performance. The test results are used to calculate measurement uncertainty which forms the basis for classification, as shown in the table below:
Classification | Uncertainty (NO2, CO and SO2) | Uncertainty (O3) | Uncertainty (benzene) |
Class 1 – Indicative | < 25% | < 30% | < 30% |
Class 2 – Objective Estimation | < 75% | <75% | < 100% |
Class 3 | < 200% | < 200% | < 200% |
The Indicative and Objective Estimation classifications are directly relatable to the descriptions provided in the EC Air Quality Directive 2008/50/EC. i.e.
- Class 1 – Indicative performance offers the highest level of certainty. Sensors that produce data in this banding are comparable in quality to NO2 diffusion tubes.
- Class 2 – Objective Estimation contains a broader range of uncertainty. Sensors that produce data in this banding could be used, for example, in identification of pollution hotspots and traffic management studies.
- Class 3 – contains the broadest margin of uncertainty that is within acceptable limits for pollution monitoring. Data in this banding is only suitable for citizen science projects.
Due to its importance in air quality monitoring assessments, most interest for gas sensors will be focussed on nitrogen dioxide (NO2). Given their relatively low concentrations in the UK, there is less interest in measuring carbon monoxide (CO) and sulphur dioxide (SO2). Local authorities typically have no requirement to measure ozone (O3) and at this point in time, there are no low-cost sensors currently available that specifically measure benzene.
Typically, uncertainties are calculated at the hourly limit concentration for NO2 and SO2, 8-hourly limit concentration for CO and O3, and annual limit concentration for benzene. All tests in the Technical Specification use hourly average data for the uncertainty calculations.
Sensor systems submitted for testing will initially undergo a basic lab test, to answer the rudimentary question “does it actually work?” to make sure the sensor will perform acceptably in subsequent tests.
Once the sensor passes this stage, the manufacturer can opt to undertake either:
- Further laboratory tests in an exposure chamber, followed by a small-scale field co-location, or
- A larger scale co-location study
Once these tests are complete, the results can be processed and compared against the performance criteria to get an indication of appropriate uses for the data produced by the sensor.
As you might expect, given the relative newness of CEN’s Technical Specification, no manufacturers have been able to complete full testing yet, so it remains extremely difficult for potential users to make an informed choice.
The good news is help is on the way, but it’ll take some time before formal sensor classification information for measuring gases is readily available.
Is the landscape any clearer for particulates?
At the time of writing this blog (June 2022), CEN continues to work on a similar Technical Specification classifications for Particulate Matter (PM). It is expected that the guidance will be released in the next 12 months. However, because of the nature of PM and the sensitivity of many sensors to humidity, testing is likely to be more problematical and agreement for the uncertainty boundaries of the various classes will be different than that for NO2, O3 and benzene.
It is likely that the different classifications will break down as follows:
Classification | Uncertainty (PM10 and PM2.5) |
Class 1 – Indicative | < 50% |
Class 2 – Objective Estimation | < 100% |
Class 3 | < 200% |
There is still a lot of discussion about exactly how to test PM sensors. It is not yet clear how to decide which detailed lab tests, field equivalence tests, location types, meteorological operation ranges and duration of tests will provide sufficiently robust performance results.
In the UK, manufacturers currently have the option to submit PM analysers for certification under the current MCERTS indicative scheme. This test programme is less exhaustive, but is expected to be updated to mirror the CEN TS, once this is published.
Test programmes and results
Inevitably, the scope of the test programme will impact on the price of testing. The requirement for lab tests, field tests in different locations and a range of environment types will have a significant cost implication, at least initially, while the amount of testing that may be required and the calculation of results are critically assessed and refined. The complexity of testing also means that there will be a fair delay until results are published.
Fortunately, Ricardo’s air quality team already have a reasonable idea of how a few sensor systems perform in the field. This helps us to determine which systems could fulfil our objectives for data quality. The table below shows (anonymously) how systems already tested by Ricardo might stack up against the CEN classification system. They are broken down per pollutant, and presented as performance out of the box (i.e. with no QA/QC applied) and performance after data correction. Green shading represents good performance. Yellow represents the next level down, followed by orange and finally red, representing the worst performance.
From this matrix, it’s clear that price and data quality are not always linked. The cheapest device in this list performs well out of the box for PM, whilst one of the most expensive performs poorly without post-processing.
The challenge here for users is the balance between price, data quality and the amount of QA/QC and data correction required to turn raw data into something usable. As shown in the matrix, some systems require just a little extra effort to produce data that would meet indicative status in any test programme. Others would require adjustment and regular checking to make sure the slope remains stable, while some are just beyond salvation!
So, what does this mean for potential users who are keen to make an informed decision?
At this point, the development of classification standards for sensor performance is still in its infancy and the lead times for results for early testers is such that those looking to invest in sensors prior to 2023 will have to do their own due diligence when it comes to procurement.
To save time and long-term costs (and your sanity) in the interim, advice from a monitoring specialist can help users to navigate the procurement process more easily, selecting the best option for their needs. Our experts routinely provide general advice about the suitability of specific sensor systems and the level of QA/QC required to fulfil user objectives.
The gold standard for processing sensor data still relies mainly on conventional thinking for air quality monitoring QA/QC i.e.
- Compile raw data, preferably in a large chunk on an ongoing basis (monthly, three monthly, etc)
- “Calibrate” the outputs, using a raft of different available information - including periodic co-location of sensors and reference monitors.
- Process the data and publish it after all the QC has been performed.
This process was successfully developed, and has been continually refined, by Ricardo for over 30+ years to deliver reference equivalent air quality monitoring results for national and local networks. There is no doubt that this method can be applied to deliver indicative or perhaps even “near-reference” results from small or medium sized local networks of sensors. However, the downside with this approach is that it is both time consuming and retrospective in nature.
As interest develops in more “hyperlocal” monitoring networks with perhaps many hundreds of sensors across a local area, it would be far more convenient to have reliable near real-time data available, to provide input to mapping and AQ management strategies. We’ll provide thoughts for how this might be achieved in a future blog...
In the meantime, if you would like advice on how to approach procuring and operating sensors for a particular project or application, please do get in touch.